Jeremy Freeman
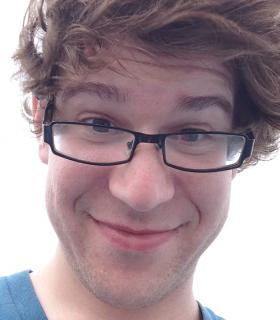
Jeremy Freeman is a neuroscientist who uses computation to understand the brain. He obtained his BA from Swarthmore College in math, biology, and psychology, and completed a PhD in neural science at New York University. Currently at HHMI's Janelia Farm Research Campus, Freeman develops new approaches for analyzing, visualizing, and manipulating large-scale patterns of neural activity in animals — flies, fish, and mice — while they perform complex behaviors. He hopes to reveal principles according to which all brains function, including our own.
-
主题演讲: Mapping the brain at scale
Abstract: Complex animal behavior reflects computation across the entire nervous system, involving the coordinated activity of thousands of neurons within and across multiple brain areas. New technologies are making it possible to monitor neural function during behavior at unprecedented scales. But the massive size and complexity of the resulting data sets has become a major bottleneck for progress. We present a solution in the form of a library, called Thunder, for fast, interactive, exploratory analysis of large-scale neural data built on the new open-source Apache Spark platform for distributed computing. Our analyses perform in minutes complex, iterative computations that would be intractable or impossibly slow with other methods. We use these analyses to characterize large-scale neural correlates of both sensory and motor behavior in the larval zebrafish, revealing both functional organization and neuronal dynamics at the scale of entire brains, and the resolution of single neurons. The open-source analytical framework we offer thus holds promise for turning brain activity mapping efforts into biological insight.
-
演讲主题: Building a platform for large-scale neuroscience
所属专题:Abstract: Neuroscience is quickly entering the realm of big data. New technologies for monitoring neural activity are yielding large, complex, high-dimensional data, in some cases reaching 1 or 10 TB per experiment. These data demand entirely new approaches to analysis. The open-source platform Spark is particularly well-suited to analyzing neural data, because it supports iterative computation, it enables interactive, exploratory analysis, and it has a powerful, elegant API that streamlines the development of complex analyses. I will show how we have adapted Spark to the demands of neural data. I will introduce the library we have developed, Thunder, and describe how it implements several large-scale analyses suitable for neural data. I will also discuss ongoing challenges, including strategies for visualization, the development of a standardized format for neural data, and the extension of our analyses to the real-time, streaming setting. Together, our work paves the way towards a general-purpose, open-source framework for large-scale neuroscience.